In the ever-changing landscape of the insurance industry, one thing remains constant: the need for accurate risk assessment and management. For centuries, actuaries have been responsible for this task, using statistical models and historical data to predict future events and set premiums. However, with the rise of big data and advanced analytics, a new player has entered the game – actuarial data science. This fusion of tradition and innovation is revolutionizing the way insurance companies operate and paving the way for a more efficient and customer-centric approach. In this article, we will delve into the world of actuarial data science, its applications, challenges, and future trends.
What is Actuarial Science?
Actuarial science is a branch of mathematics that uses mathematical and statistical techniques to assess financial risks and uncertainties. It involves evaluating the probability of future events and their financial impacts on individuals or organizations. The primary goal of actuarial science is to help insurance companies determine appropriate premiums, reserves, and other financial strategies to manage risk effectively.
Actuaries are highly skilled professionals who possess a deep understanding of mathematics, statistics, finance, and economics. They analyze data and build models to evaluate potential risks, such as death, illness, accidents, and natural disasters. These risk assessments are then used to develop insurance products and set premiums that accurately reflect the level of risk involved.
What is Data Science?

Data science is an interdisciplinary field that combines various scientific methods, processes, algorithms, and systems to extract insights and knowledge from data. It involves collecting, organizing, and analyzing large volumes of data to identify patterns, trends, and correlations. Data scientists use a combination of statistical methods, machine learning, and programming skills to extract meaningful insights from data and make data-driven decisions.
The main difference between actuarial science and data science lies in their objectives. While actuarial science focuses on assessing risk and predicting future events, data science goes beyond that by uncovering hidden patterns and insights in data that may not be immediately apparent. Additionally, data science encompasses a broader range of techniques and tools, making it more versatile in handling different types of data.
Importance of Actuarial Science
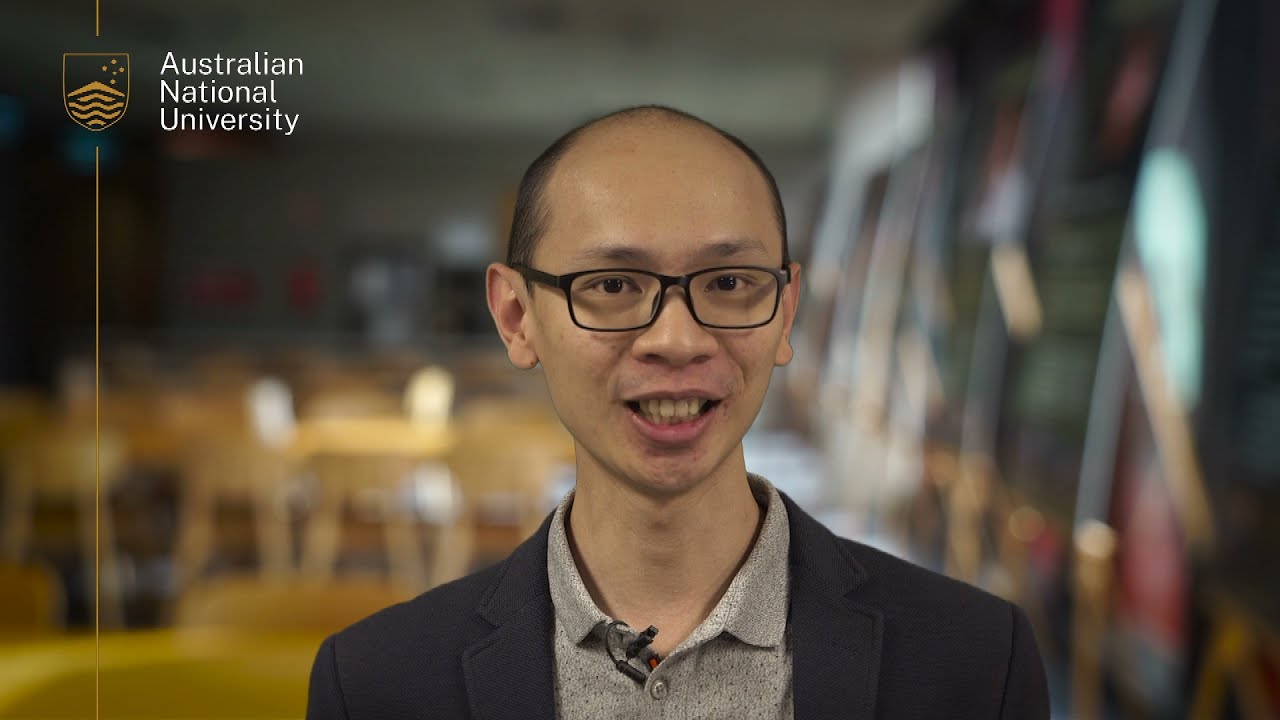
Actuarial science plays a vital role in the insurance industry as it helps insurers mitigate risks and remain financially stable. By accurately assessing risks, actuaries can help insurance companies set appropriate premiums and reserves, ensuring that they have enough funds to pay out claims without going bankrupt. This function is especially critical for life insurance companies, where long-term financial planning is crucial for their sustainability.
Moreover, actuarial science enables insurance companies to develop new products and services that cater to the evolving needs of customers. For instance, with the rise of the gig economy, there has been an increasing demand for insurance coverage for freelancers and part-time workers. Actuaries can use data analysis to assess the risks associated with this segment and design insurance products tailored to their needs.
Importance of Data Science
The rise of big data has brought data science to the forefront, making it a crucial aspect of decision-making in various industries, including insurance. With the explosion of data from various sources, such as social media, IoT devices, and wearables, it would be impossible for actuaries to manually analyze and make sense of it all. Data science not only allows them to handle large volumes of data but also provides the tools and techniques to uncover hidden patterns and trends.
Data science is also essential in modeling complex risks that traditional actuarial methods may struggle to capture. For instance, natural catastrophes, such as hurricanes and earthquakes, are becoming increasingly frequent and severe, making it difficult to rely solely on historical data for risk assessment. Data science techniques, such as predictive analytics, can help insurers incorporate external data sources, such as weather patterns and topographical data, to improve risk modeling.
Intersection of Actuarial Science and Data Science
Actuarial science and data science may seem like two distinct fields, but in reality, they complement each other. While actuarial science brings the mathematical foundations and domain expertise, data science provides the necessary tools and techniques to analyze and extract insights from massive amounts of data. Together, they form a powerful force that can transform the way insurance companies operate.
The primary application of actuarial data science is in risk assessment and management. By combining traditional actuarial models with advanced data analysis techniques, actuaries can develop more accurate risk assessments and pricing models. This enables insurance companies to price their products more competitively, leading to better customer satisfaction and retention.
Another prominent application of actuarial data science is in fraud detection. Insurance fraud costs the industry billions of dollars every year, making it essential for insurers to have robust fraud detection systems in place. By leveraging data science techniques, such as anomaly detection and predictive modeling, insurance companies can identify suspicious patterns and behaviors and prevent fraudulent claims from slipping through the cracks.
Moreover, actuarial data science has enabled insurers to personalize their offerings and provide more customized solutions to customers. With data analytics, insurers can gain valuable insights into customer behavior, preferences, and needs, allowing them to tailor their products and services accordingly. This not only helps in attracting new customers but also increases customer loyalty and retention.
Applications of Actuarial Science and Data Science
The applications of actuarial data science are vast and extend beyond just risk assessment and management. Let’s take a closer look at some of its other use cases in the insurance industry.
Marketing and Sales
Data science plays a crucial role in marketing and sales for insurance companies. By analyzing customer data, insurers can identify which marketing strategies are most effective in attracting and retaining customers. They can also use data science techniques to segment their customer base and target specific demographics with personalized messaging.
Underwriting
Underwriting is the process of evaluating an individual’s risk profile to determine whether they should be insured and at what rate. Data science has made this process more efficient by automating many of the manual tasks involved in underwriting. With the help of machine learning algorithms, insurance companies can analyze large amounts of data and make accurate underwriting decisions quickly.
Claims Processing
Data science has revolutionized the claims processing function in the insurance industry. By using predictive analytics, insurers can assess the probability of a claim being fraudulent or legitimate, expediting the processing of genuine claims and detecting fraudulent ones. This not only saves time and resources but also improves the overall customer experience.
Challenges and Future Trends
While actuarial data science brings immense benefits to the insurance industry, it also faces numerous challenges that need to be addressed. One of the most significant challenges is data quality. With the increasing volume of data sources, ensuring the accuracy and validity of data becomes critical. Inaccurate or incomplete data can lead to incorrect risk assessment and poor decision-making, ultimately resulting in financial losses for insurance companies.
Another challenge is the shortage of skilled professionals who possess both actuarial and data science expertise. As the demand for actuarial data scientists continues to rise, there is a growing need for individuals with a deep understanding of both fields. To address this issue, universities and training institutions are starting to offer specialized programs that bridge the gap between actuarial science and data science.
Looking into the future, we can expect actuarial data science to become even more vital in the insurance industry. With the rapid advancements in technology and the increasing availability of data, the potential for innovation is endless. We can expect to see more sophisticated data analysis techniques, such as natural language processing and image recognition, being incorporated into risk assessment and management processes.
Additionally, as the concept of connected devices and the Internet of Things (IoT) becomes more prevalent, we can expect to see a proliferation of data from these sources. This will open up new opportunities for insurers to develop more personalized and dynamic insurance products based on real-time data.
Conclusion
The rise of actuarial data science is reshaping the insurance industry in unprecedented ways. By combining traditional actuarial expertise with advanced data science techniques, insurance companies can better understand and manage risks, make more informed decisions, and provide enhanced customer experiences. As we move towards a more data-driven future, it is clear that actuarial data science will continue to play a crucial role in shaping the insurance landscape.