In today’s digital age, data has become the new currency. The amount of data generated and stored by businesses is increasing at an exponential rate. This data holds valuable insights that can drive strategic decisions and fuel innovation. However, the challenge lies in effectively utilizing this vast amount of data to gain meaningful insights. This is where the role of a data science architect becomes crucial.
A data science architect is a strategic visionary who bridges the gap between raw data and actionable insights. They are responsible for designing, building, and maintaining the entire data science ecosystem within an organization. In this article, we will delve deeper into the responsibilities, skills, and challenges of a data science architect, and how they drive innovation and success in businesses.
Overview of Data Science Architect Role
The term “data science” has gained immense popularity in recent years, and with it, the demand for data scientists and data science architects has also increased. While both roles are essential for leveraging data, there are key differences between them.
Data scientists focus on building models and algorithms to extract insights from data. On the other hand, data science architects take a more holistic approach, understanding the business needs and creating a framework for data utilization. Their role goes beyond coding and involves managing the entire data science lifecycle, including data acquisition, storage, processing, and analysis.
A data science architect must have a combination of technical expertise, business acumen, and communication skills to be successful in their role.
Technical Expertise
At its core, data science is a highly technical field. Therefore, a data science architect must have a strong foundation in various technical areas such as data engineering, cloud computing, data warehousing, and machine learning. They need to understand the intricacies of data acquisition, processing, storage, and analysis to design efficient and scalable systems.
Furthermore, with the rise of big data and cloud computing, a data science architect must also be well-versed in distributed systems and parallel computing. This knowledge is essential for handling large volumes of data and achieving faster processing speeds.
Business Acumen
While technical skills are crucial, a data science architect must also have a deep understanding of the business they work for. They need to align their data strategy with the company’s goals and objectives. This involves collaborating closely with different business units to understand their needs and identify opportunities where data can drive value.
Moreover, the architect must be able to translate business requirements into data-driven solutions. This requires them to have a solid understanding of various industries and how data can be utilized to solve specific problems.
Communication Skills
A data science architect must be able to communicate complex technical concepts to non-technical audiences effectively. They act as a liaison between the data team and other departments within the organization. Therefore, they need to be able to explain the importance and impact of data-driven projects in a way that is easy for others to understand.
Clear communication is also crucial when working with stakeholders and managing expectations. The architect must be able to convey the potential challenges and limitations of a project and propose alternative solutions.
Importance of Innovation in Businesses

Innovation has always been a driving force behind the success of businesses. In today’s fast-paced and competitive market, the ability to innovate has become even more critical. Companies that fail to innovate risk becoming obsolete or losing ground to their competitors.
So, what exactly is innovation? Innovation is not just about coming up with new ideas; it’s about implementing these ideas and creating value. It is about finding new ways to solve problems, streamline processes, and improve products and services. And in this age of data, one cannot ignore the role of data science in driving innovation.
Data science offers companies the opportunity to gain a deeper understanding of their customers, products, and operations. With the help of data, businesses can identify patterns and trends, make predictions, and uncover hidden insights. These insights can then be used to develop innovative products, services, and strategies that meet the changing needs of customers and stay ahead of the competition.
How Data Science Architect Drives Innovation
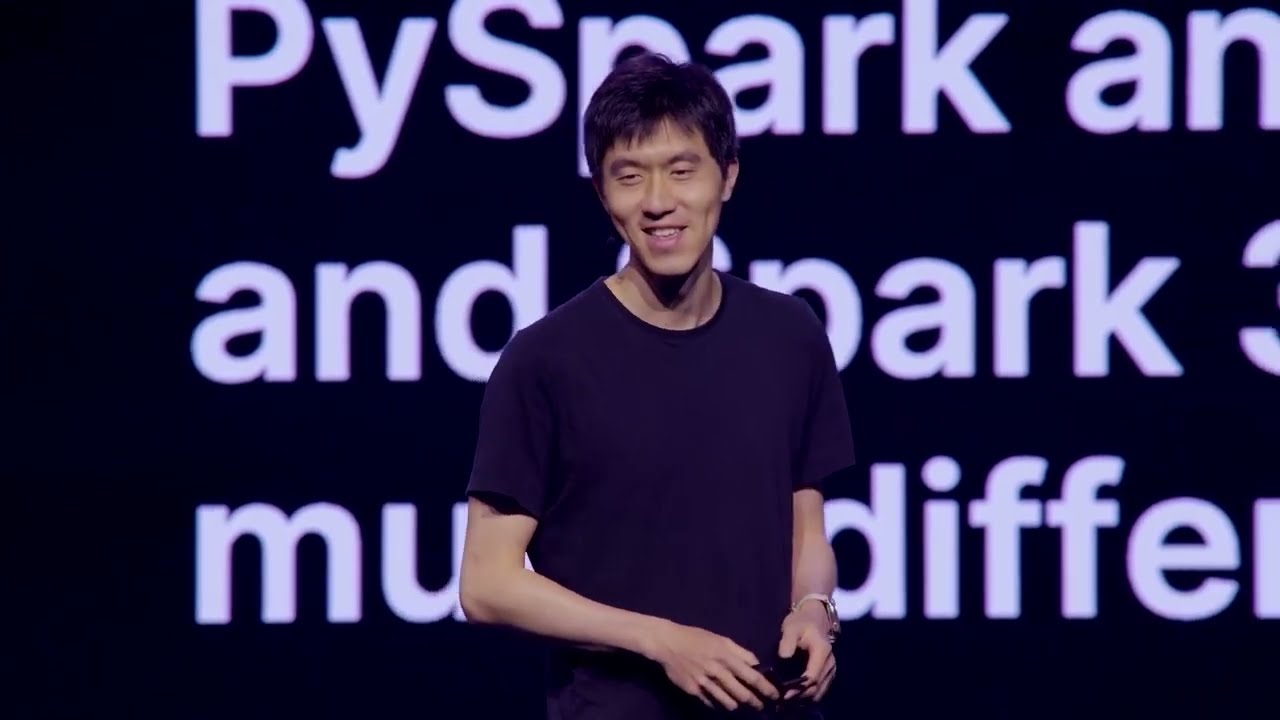
The role of a data science architect is crucial in driving innovation within an organization. They are responsible for creating a cohesive data strategy that aligns with the company’s goals and objectives. Here are some ways in which a data science architect drives innovation:
Identifying Opportunities for Data Utilization
One of the primary responsibilities of a data science architect is to understand the business goals and identify areas where data can be utilized. They work closely with different departments to gather insights into their processes, pain points, and opportunities. This allows them to identify use cases where data analysis can bring about significant improvements.
For example, in the retail industry, a data science architect might identify an opportunity to use customer purchasing history and demographic data to create targeted marketing campaigns. By leveraging data, the company can personalize its messaging and offers, leading to higher customer retention and increased sales.
Creating Efficient Data Infrastructure
Data science projects require large volumes of data to be processed and analyzed. Therefore, having an efficient data infrastructure is essential for the success of these projects. A data science architect designs data pipelines that efficiently handle data ingestion, storage, and processing. They also ensure that the infrastructure is scalable and can accommodate the ever-increasing amount of data.
With a robust data infrastructure in place, data scientists can focus on building models and extracting insights from data, rather than worrying about data management.
Applying Advanced Analytics Techniques
Data science architects are well-versed in various analytics techniques such as machine learning, natural language processing, and data mining. They leverage these techniques to extract meaningful insights from data. With the help of advanced analytics, companies can unlock patterns and trends that are not easily identifiable through traditional methods.
For instance, a data science architect in the healthcare industry might use natural language processing to analyze patient records and identify patterns that could indicate potential health risks. This information can then be used to develop personalized treatment plans and improve patient outcomes.
Success Stories of Companies with Data Science Architects
Numerous companies across various industries have leveraged data science architects to drive innovation and achieve success. Here are some examples of how these companies have utilized data to their advantage:
Netflix
Netflix is a prime example of how data-driven insights can transform a company’s operations. The streaming giant employs a team of data scientists and data science architects who analyze user data to personalize movie recommendations. With over 200 million users globally, Netflix collects a wealth of data on viewing habits, ratings, and searches. This data is then used to create personalized content recommendations, resulting in higher customer satisfaction and increased retention rates.
Moreover, Netflix utilizes data to make strategic decisions about its original content. By analyzing audience preferences and viewing patterns, they can identify which genres and themes are most popular and create content accordingly.
Amazon
Amazon, the world’s largest online retailer, uses data science at every step of the customer journey, from browsing to purchasing. The company employs a team of data science architects who are responsible for creating sophisticated algorithms that power personalized product recommendations, search results, and pricing strategies.
In addition, Amazon also uses data science to optimize its supply chain and inventory management systems. By analyzing data on customer demand and sales trends, they can ensure that products are available when and where customers need them, leading to higher customer satisfaction and increased revenue.
Challenges Faced by Data Science Architects
While the role of a data science architect is vital, it comes with its fair share of challenges. Here are some common challenges faced by data science architects:
Managing Multiple Stakeholders
Data science projects involve multiple stakeholders, including business leaders, data scientists, IT teams, and executives. Each of these stakeholders has different expectations and priorities, which can sometimes be conflicting. Managing these stakeholders and ensuring that everyone is on the same page can be challenging.
Data science architects must effectively communicate with all stakeholders, manage their expectations, and ensure that the project remains aligned with the organization’s goals.
Ensuring Data Quality and Governance
The success of any data-driven project depends on the quality of the data used. Data scientists rely on high-quality, accurate, and relevant data to build models and extract insights. However, ensuring data quality is not an easy task. Data may be scattered across various systems, in different formats, and may be subject to errors and biases.
Moreover, with the increasing emphasis on data privacy and security, data governance has become a crucial aspect for organizations. A data science architect must ensure that proper data governance policies and procedures are in place to protect sensitive data and comply with regulations.
Technical Challenges
As mentioned earlier, data science is a highly technical field, and new tools and techniques are constantly emerging. This makes it challenging for data science architects to stay updated with the latest trends and technologies.
Moreover, with the rise of big data, cloud computing, and AI, data science projects have become increasingly complex. Architecting efficient, scalable, and secure systems that can handle large volumes of data is no easy feat. It requires a deep understanding of various technical areas, and architects must constantly learn and adapt to new technologies to keep up with the pace of innovation.
Strategies for Success in the Role
Becoming a successful data science architect takes more than just technical skills. Here are some strategies that can help you excel in this role:
Develop a Business Mindset
To be successful as a data science architect, you must have a solid understanding of the business you work for. This means learning about its goals, objectives, processes, and products. This knowledge will help you identify opportunities for data utilization and design solutions that align with the company’s vision.
Moreover, understanding business concepts such as ROI, cost-benefit analysis, and risk management will help you communicate effectively with stakeholders and justify the use of resources for data science projects.
Keep Learning
The field of data science is constantly evolving, and to stay ahead, you must keep learning. Attend conferences, workshops, and webinars to gain insights into the latest trends, tools, and techniques. Networking with other data professionals and exchanging ideas can also help you stay updated with industry developments.
Additionally, take advantage of online resources such as MOOCs (Massive Open Online Courses) and blogs to learn new skills and deepen your understanding of various technical areas.
Hone Your Communication Skills
As a data science architect, you will have to communicate with different stakeholders regularly. This could include non-technical team members, executives, or clients. Therefore, it is essential to hone your communication skills to clearly convey complex technical concepts in a way that is easy for others to understand.
Practice creating visualizations and presentations that effectively communicate insights from data. Additionally, develop active listening skills to understand the needs and concerns of stakeholders better.
Conclusion
The role of a data science architect is vital in today’s data-driven world. They are the strategic visionaries who bridge the gap between raw data and actionable insights. A successful data science architect must have a combination of technical expertise, business acumen, and communication skills.
They play a crucial role in driving innovation within organizations by identifying opportunities for data utilization, designing efficient data infrastructure, and applying advanced analytics techniques. Companies such as Netflix and Amazon have successfully leveraged data science architects to achieve success and stay ahead of the competition.
However, the role is not without its challenges. Managing multiple stakeholders, ensuring data quality and governance, and keeping up with the constantly evolving technical landscape are some of the common challenges faced by data science architects.
To excel in this role, it is essential to develop a business mindset, keep learning, and hone your communication skills. With the right combination of skills, a data science architect can drive innovation and help their organization achieve success in today’s data-driven world.